这篇论文提出了一种基于Transformer的时序行为检测方法TadTR。TadTR引入了集合预测框架,极大地简化了时序行为检测的流程。该方法能直接将少量可学习的行为查询映射为完备、不重复的行为实例,去除了锚片段设计、后处理等经验式设计,方法的各个模块集成在单个完全可微分的神经网络中。TadTR相比先前性能最好的方法速度提升10倍,且在THUMOS14、ActivityNet和HACS Segments上取得了单网络方法的最佳性能。
This paper proposes a Transformer-based temporal action detection method called TadTR. It introduces the set prediction framework, which significantly simplifies the pipeline of temporal action detection. It directly maps a small set of learned action queries into complete and unique action instances, eliminating hand-crafted components such as anchor setting and post-processing. All modules are integrated into a fully differentiable neural network. TadTR runs 10 times faster than the previous state-of-the-art method and achieves the best performance of single-network methods.
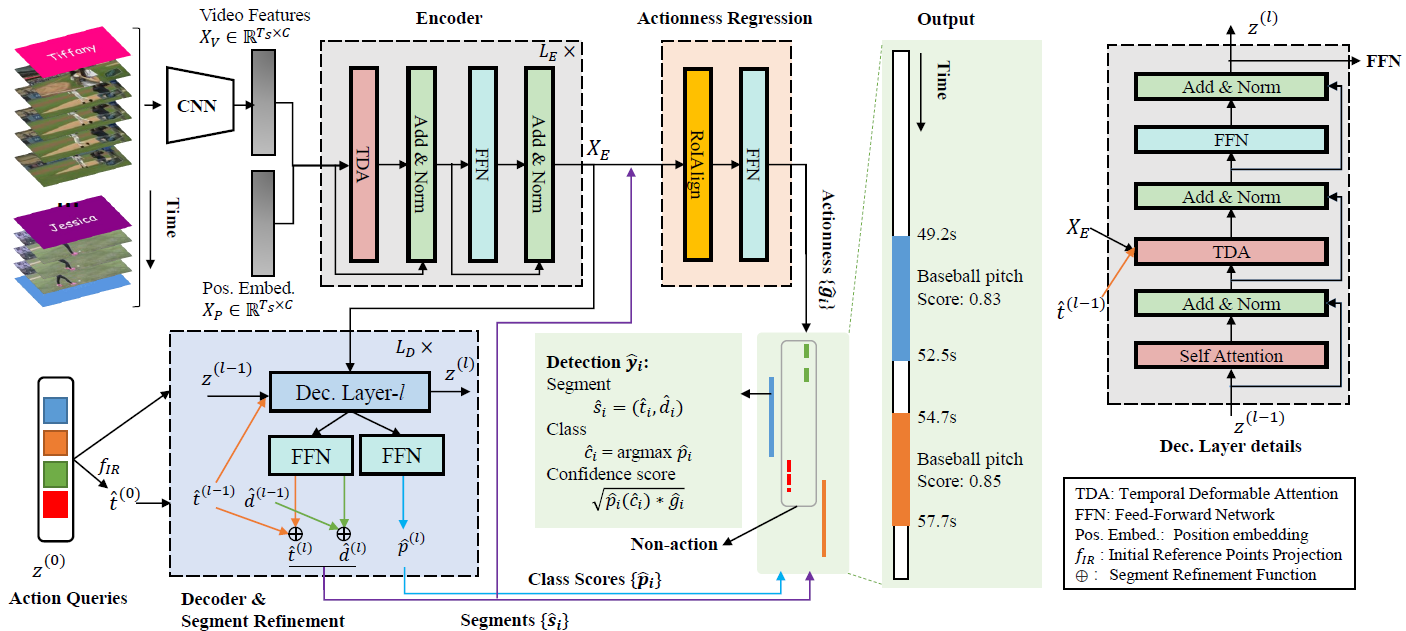
论文链接:https://arxiv.org/abs/2106.10271
代码:https://github.com/xlliu7/TadTR