近年来,视频实例分割(VIS)在很大程度上是由离线模型推动的,而在线模型通常比同时代的离线模型相差很多,这是一个很大的缺点。 通过剖析当前的在线模型和离线模型,改论文证明了性能差距的主要原因是容易出错的帧间跟踪,并基于对比学习提出了 IDOL,它在三个基准上优于所有在线和离线方法。
In recent years, video instance segmentation (VIS) has been largely advanced by offline models, while online models are usually inferior to the contemporaneous offline models by over 10 AP, which is a huge drawback. By dissecting current online models and offline models, we demonstrate that the main cause of the performance gap is the error-prone association and propose IDOL based on contrastive learning, which outperforms all online and offline methods on three benchmarks.
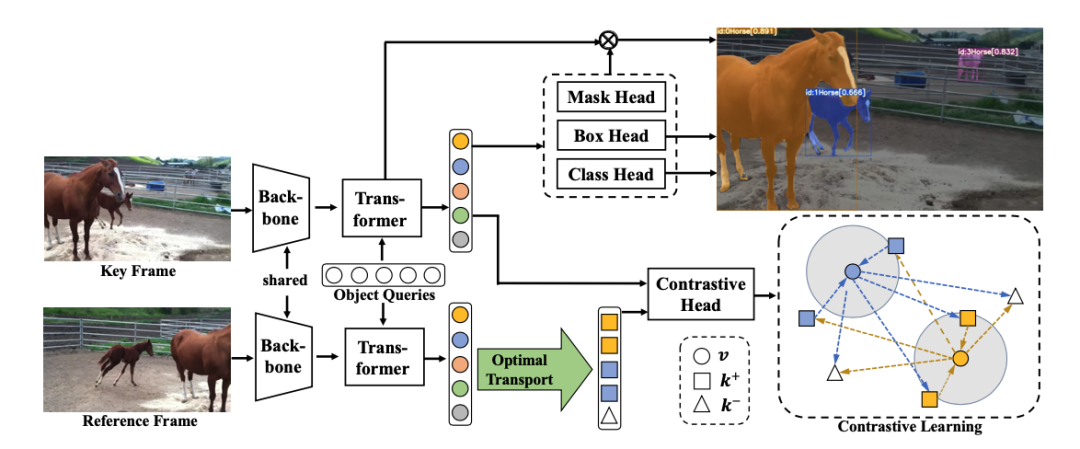
论文链接:https://arxiv.org/abs/2207.10661
代码:https://github.com/wjf5203/VNext